Artificial Intelligence Speeds the Search for Stable Proteins Used in Drug Development, Biofuels and Food Production
Researchers develop machine-learning model to identify chemical compounds most likely to stabilize desired proteins
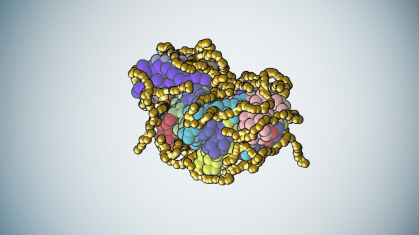
Harnessing the power of robotics and machine intelligence, researchers from Rutgers University and Princeton Engineering have found a way to design stable proteins in a fraction of the time it historically took to stabilize enzymes which are used in various applications including dietary supplements, diagnostics, cleaning products, and biofuel and food production.
“Enzymes are used in a wide variety of commercial, medicinal and industrial applications,” said Adam Gormley, an assistance professor of biomedical engineering at Rutgers University–New Brunswick. “In each application, extensive efforts are made to stabilize the enzyme to retain activity in harsh environments. Our platform approach provides a new opportunity to accelerate stabilization efforts across all these applications.”
Led by Gormley and Michael Webb of Princeton University, the researchers published their findings in the journal Advanced Materials.
Stabilizing proteins is a challenge for those doing research in drug creation, biofuel production and plastic recycling. The conventional approach uses trial and error – estimating which chemical compounds will stabilize proteins under different conditions – which can take months and is never guaranteed to work.
In the new system, engineers use a machine-learning model to identify chemical compounds most likely to stabilize desired proteins. The model helps narrow hundreds of thousands of possibilities to a few likely candidates. A robotic assembly platform then produces samples of the molecules for evaluation. Combining the robotic platform with the machine-learning model turns out results in as little as a few days.
Because of its ability to churn through vast amounts of data, the machine-learning model often recommends candidate molecules – ones that will help quickly stabilize the enzymes – that wouldn’t have occurred to scientists.
In developing the system, the team turned to three proteins with distinctive properties, including one found in horseradish that is used in hospitals as a biosensor to detect diseases and water-treatment plants.
“The amount of time and energy this model could save scientists is extremely important,” said Gormley. “Notably, we could streamline the process and speed it further by integrating the machine-learning model with the robotics systems we have on site. It’s an exemplary approach that can be extended to other proteins.”
The research was supported by funding from the National Institutes of Health and the National Science Foundation and by the facilities of Princeton Research Computing and the U.S. Department of Energy’s Brookhaven National Laboratory and Office of Basic Energy Sciences Program.
The study was coauthored by Rutgers’ Matthew J. Tamasi, Shashank Kosuri, Heloise Mugnier, Rahul Upadhya and N. Sanjeeva Murthy as well as other researchers at Princeton.